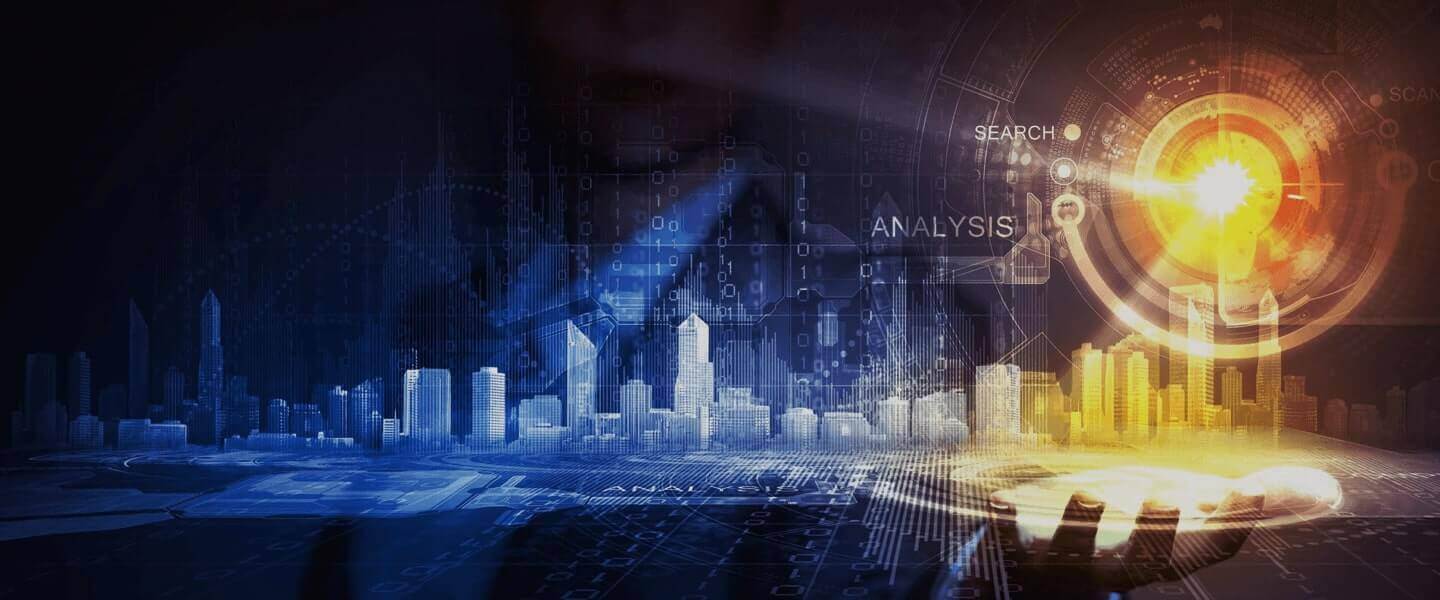
While some enterprise organizations are just beginning to dabble in AI solutions, the most innovative and future-focused among them are already adopting and executing Conversational AI solutions to transform several facets of their business.
Visionary C-Suite and senior-level executives are making connections between technology and business that others have yet to discover. The result: Conversational Enterprise.
What is Conversational Enterprise?
A Conversational Enterprise is an organization that integrates Conversational AI into their internal and external workflows. They use bots to interface with their customers, employees and the world, which results in more seamless and straightforward conversations — and better business all around.
While this definition is simple enough to understand, leading your organization into this customer-centric, operationally efficient future requires foresight and vision. CMOs, CSOs, CDOs, VPs of Digital, and other senior leaders can learn three important things from the forward-thinking executives that are carving the path today.
AI Can Be Used to Improve Organizational Efficiency
Conversational AI is all about data — but a Conversational Enterprise knows how to put that data to the best use.
Analyzing data
Conversational AI platforms equipped with machine learning tools can analyze large packets of unstructured data to identify key motivations of customers or employees based on how they are engaging with the system.
This data can come from web chats, audio calls, text messages, social media posts, or even conversations with other smart bots in the system. The insight gleaned from that data allows the organization to better predict their customers’ and employees’ wants and needs, and improve their experience.
Improving loyalty is no longer just about making customers happier — it’s about increasing employee engagement, too. Happy employees lead to happier customers, and AI data can power it all.
Understanding intent
With a Conversational AI platform that uses multiple integrations and Natural Language Understanding (NLU) engines to ensure accuracy, the organization can process the raw data from smart bots to create “intent models.” Structured, labeled data is matched to its context to help smart bots get smarter over time, and to ensure they’re learning from every conversation they have with users.
The result is a better and better experience for internal and external users, regardless of the communication channel they use.
Training AI with deep learning
Conversational Enterprise leaders use deep learning (part of machine learning) to train Conversational AI to anticipate outcomes when they receive a specific set of inputs. This deep structured learning can be both supervised and unsupervised, and is only available with the more advanced Conversational AI platforms.
Deep learning helps the organization process large sets of data like images, text, and audio recordings, then compile this data to generate insights. Those insights then get fed back into the Conversational AI platform, making it even more efficient in dealing with transactions without human intervention.
A real-life example of how this can be applied is targeting a customer with a finely tuned marketing message based on their purchase history. Another application would be enabling a chat bot to respond to highly nuanced customer queries with just the right answer.
Conversational AI Continuously Improves the Customer Experience
Becoming a Conversational Enterprise isn’t just a series of steps your organization must follow. It’s a new mentality and an understanding that continuous improvement is the only way to stay ahead. Conversational Enterprise leaders are using AI to solidify the new mentality within their organizations and drive this continuous improvement.
Conversational AI combines with other technology for better customer service
By combining Conversational AI with other established and emerging communications technologies, your organization can better predict customer needs — and better serve them.
For example, combining AI with voice recognition technology can lead to more efficiently routed calls, improved call center management, and enhanced customer experience. But it can be taken even further and used to analyze the tone of a user’s voice and determine their emotional state. Then if the user is not having a satisfactory experience, the call can get automatically rerouted to a human operator for resolution.
Or you could combine AI with customer demographic data, transaction data, and social media insights to develop more thoughtful, valuable product and service ideas. You could use this same combination to provide customers with long-tail product recommendations.
Enhancing the performance of existing analytics with neural nets
Because Conversational AI creates a neural conversational mesh around the organization, it’s even more effective in industries where data is rich, immense, and integrates human interactions. Healthcare, insurance, telecommunications, banking, retail and media are just a few examples of industries that can experience a massive improvement in analytics capabilities with AI.
In healthcare, for instance, Conversational AI can be used in disease diagnosis. By compiling and analyzing rich data sets including images, videos and MRIs, AI can provide routine medical advice to individuals who do not have easy access to medical facilities.
With further improvements in technology and better regulation of data by industries, the sky is the limit for how Conversational Enterprises can boost their performance with AI.
Facing the 3 Key Challenges to Becoming a Conversational Enterprise
Even the most prepared, most far-sighted enterprise leader encounters challenges as his or her organization evolves into a Conversational Enterprise. Here are the three main challenges we’ve seen senior leadership tackling:
-
Massive training data sets
Compiling large amounts of data to generate useful predictions and intelligent user interfaces requires a solid plan of action. This plan needs to include:
- Data acquisition strategy
- Ontology definitions and approved taxonomy
- Possibly maintaining your own data centers if there are regulatory and security concerns regarding the information captured
-
Process Implementation
To ensure smooth operations, robust data-management and maintenance processes are required — but it’s the last mile that really counts. Senior leaders need to make sure that the insights delivered by their Conversational AI platform is absorbed and embodied by the people and processes within the enterprise.
-
Insufficient workforce
Developing and maintaining a deep neural network is a complex task requiring highly skilled professionals. Unfortunately, the demand for these individuals currently outstrips the supply.
Your First Step to Becoming a Conversational Enterprise
Conversational AI is already having a massive impact on a large number of industries — and its impact is only going to grow as more enterprise leaders see the value of becoming a Conversational Enterprise.
Senior executives should move quickly and take steps towards transforming their companies into Conversational Enterprises — but this is a complex endeavor. It can be a smarter play to outsource some of the more costly capabilities and infrastructure to experts.
The best place to start, however, is to get an assessment of where your organization is today on the road to becoming a Conversational Enterprise. From there you’ll better understand the right next step to preparing your organization for the future.
Not sure where you are —really — on the road to becoming a Conversational Enterprise? Provide your details below to subscribe to our Conversational Enterprise Article Series.